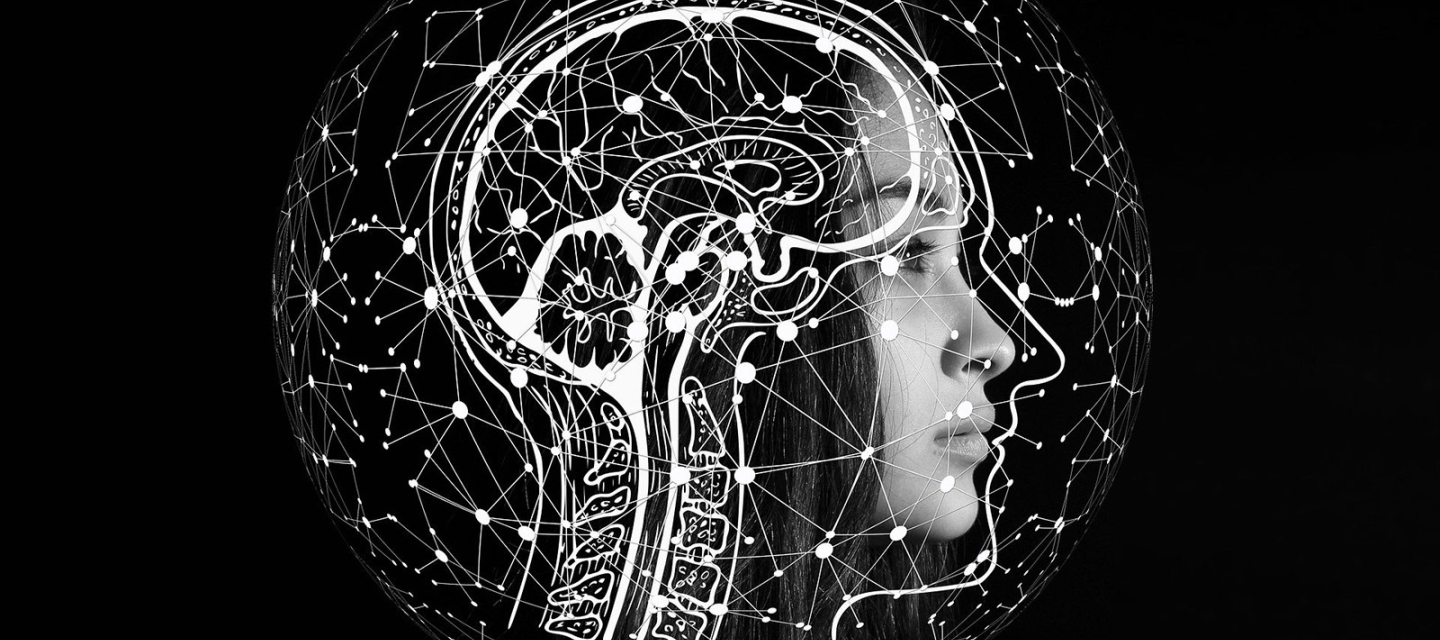
Machine Learning (ML), a subfield of Artificial Intelligence (AI) utilizes the knowledge of mathematics, statistics, and computer science to build computer algorithms for specific aims.
The algorithmic system learns from experimental or computational data, analyzes it, and builds patterns to anticipate behavior with the goal to make better judgments. It is, therefore, no surprise that over the past decade, ML has become a vital tool in all branches of STEM.
The fields of Materials Science and Device Physics have evolved in step during this time as well, allowing scientists to utilize a broad variety of model prediction methods and tools based on ML algorithms for use in different materials and devices. With the use of these tools, scientists can now devise new ways of investigating materials' characteristics and improving materials' performance in general. Generating data from a specific process or device followed by data wrangling, feature generation, feature engineering, constructing models, and eventually making choices to get optimum outputs are the steps in the ML processes. The end goal of this endeavor effectively is to lead to what is termed as ‘Accelerated Discovery’ for any given research avenue or technology.
Dr. Ahmed and Dr. Carbonara from the center, in collaboration with faculty and students at Bangladesh University of Engineering and Technology (BUET), UB, RPI, and SUNY Poly, have utilized Accelerated Discovery in investigating non-toxic components for implementation in next-generation solar technology. The motivation for this successful work (aspects of which are still ongoing) centered around assessing the perovskite-on-silicon tandem solar technology that is imminently intersecting global Clean Energy markets. Is the technology going to harm the environment, affect ecology, and ultimately have ironic detrimental impacts on humans? By identifying toxic device components, understanding the economic viability of the technology, and using accelerated discovery, we have proposed alternate materials that are safer and environmentally friendly, yet give comparable device efficiency numbers to suggested traditional toxic materials.
Dr. Ahmed, together with collaborators, is additionally utilizing Accelerated Discovery to probe and determine ideal features and characteristics for non-Li ion alternatives, along with various exotic and disruptive electrode materials, for both batteries as well as supercapacitor applications.
Collaborative Projects within the Center
- Through CISNN’s collaborative activities, Dr. Ahmed will collaborate with Dr. Pathak and Dr. Biswas to understand the synthesis and deposition of ML-derived optimum compositions of materials (for applications in solar, battery and supercapacitor applications). This will be followed by device fabrication and testing.
- Dr. Ahmed will collaborate with Dr. Qian and Dr. Hunter to explore economic viability models of the various technologies and carry out comparisons against the traditional fossil fuel (for solar) and Li-ion (for battery/supercapacitor) technologies.
- Lastly, Dr. Ahmed will collaborate with Dr. Shauku to critically understand and delineate the Ethical, Legal, and Social Implications (ELSI) of manifesting the various versions within the highlighted solar/battery/supercapacitor technologies, taking into consideration the materials that are being used, and the impact of such technologies against the current industrial standards.
The conflux of ethics with device physics and Accelerated Discovery is best illustrated through a recent publication between Dr. Ahmed, Dr. Carbonara, and collaborators. This work was borne out of ethical considerations for the next-generation solar technology, where environmentally unfriendly components are used as they provide enhanced device efficiency, stability, and low degradation. In this work, PIs investigated the device physics of non-toxic components utilizing advanced Machine Learning algorithms to provide compositions (that were otherwise near impossible to identify and fabricate) that will provide comparable device performance with their toxic counterparts.
Inset: The left side of the image below is the ‘Explanatory’ component of the research conductive—it provides the context, motivation, and methodology of the work. The right side of the image is the ‘Predictive’ component of the research, where the predictions and insights gleaned from the Machine Learning models were used to computationally develop the devices, simulate them, and obtain characteristic outputs that allowed the researchers to gauge their performance and do comparative studies against industrial standard data.
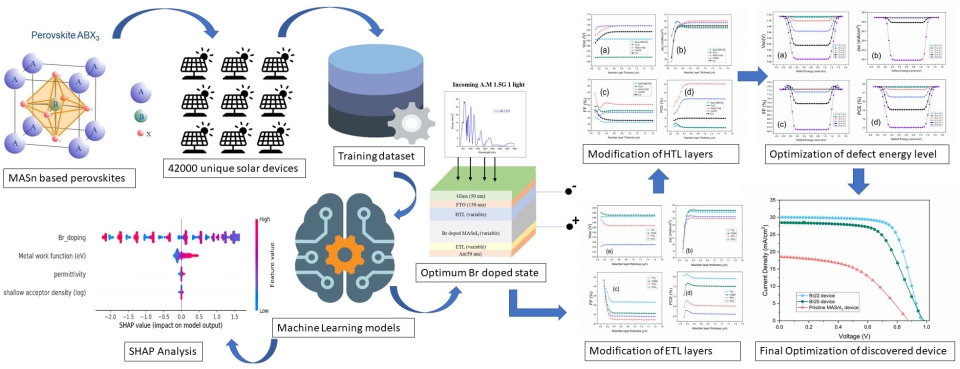
Reference: Md Shafiqul Islam, Md Tohidul Islam, Saugata Sarker, Sadiq Shahriyar Nishat, Md Rafsun Jani, Abrar Rauf, Hasan Al Jame, Sumaiyatul Ahsan, Kazi Md Shorowordi, Harry Efstathiadis, Joaquin Carbonara, Saquib Ahmed* “A Machine Learning Approach to Delineate Impact of Materials Properties on Solar Cell Device Physics”, ACS Omega, accepted May 2022.